As a more direct use of the TROPOMI satellite data and to improve its societal relevance, a spatial downscaling approach has been developed and applied. The method increases the spatial resolution of the data significantly from the original ca. 5.5 km x 3.5 km TROPOMI footprint of the Level-2 data to significantly higher resolution on the order of hundreds of meters, depending on the used spatial proxy dataset.
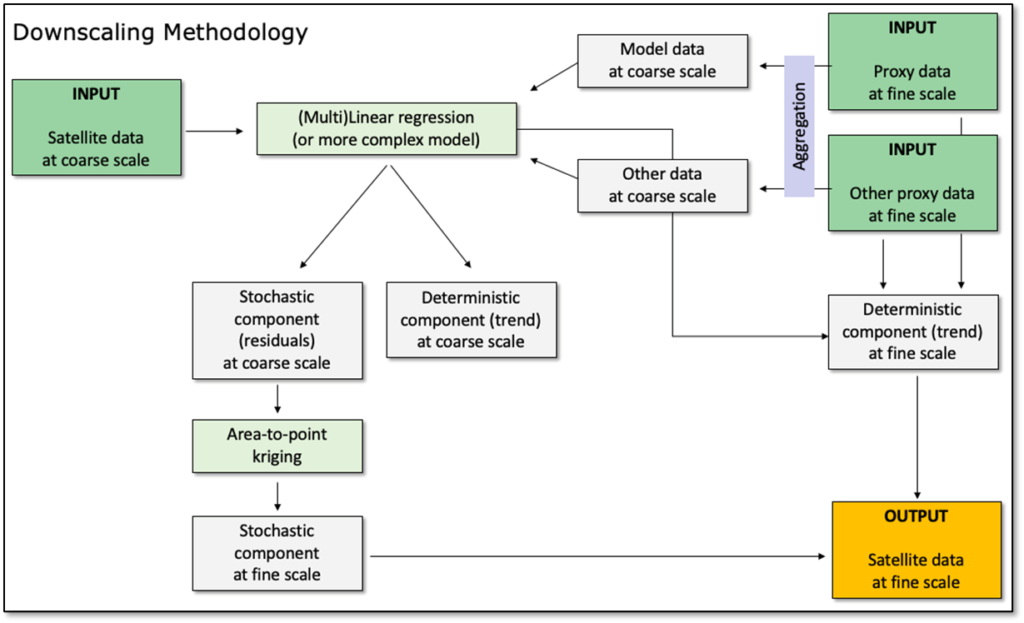
The methodology is based on geostatistics and is described in more detail Stebel et al. (2021) (see concept illustration above). It is essentially increasing the spatial resolution of a coarse-resolution source dataset (in our case satellite data of air quality) with the help of spatial proxy or auxiliary datasets that are available at a fine spatial resolution and that are, to some extent, correlated with the source dataset. As such, the technique makes use of the assumption that the spatial patterns of the unknown fine-scale field of the source variable will be similar to the spatial patterns of the fine-scale proxy datasets. In simplified terms, the various input datasets are brought to the same coarse resolution and a statistical model is fitted to directly relate the satellite and the proxy data. The model choice is arbitrary and could range from simple linear regression models (used here) to more advanced non-linear models such as random forest or similar. Subsequently, the spatial residuals from the model are calculated, downscaled to the fine target resolution using area-to-point kriging (Kyriakidis, 2004), and added to the deterministic trend component of the fitted model.
Based on this method, we first derive surface NO2 from the S5P/TROPOMI tropospheric column densities and then downscale these to 500 m spatial resolution using data from the EPISODE dispersion model (Hamer et al. 2020) as a spatial proxy. The following figure shows an example for a TROPOMI orbit on 2021-02-22 over the city of Warsaw, Poland.
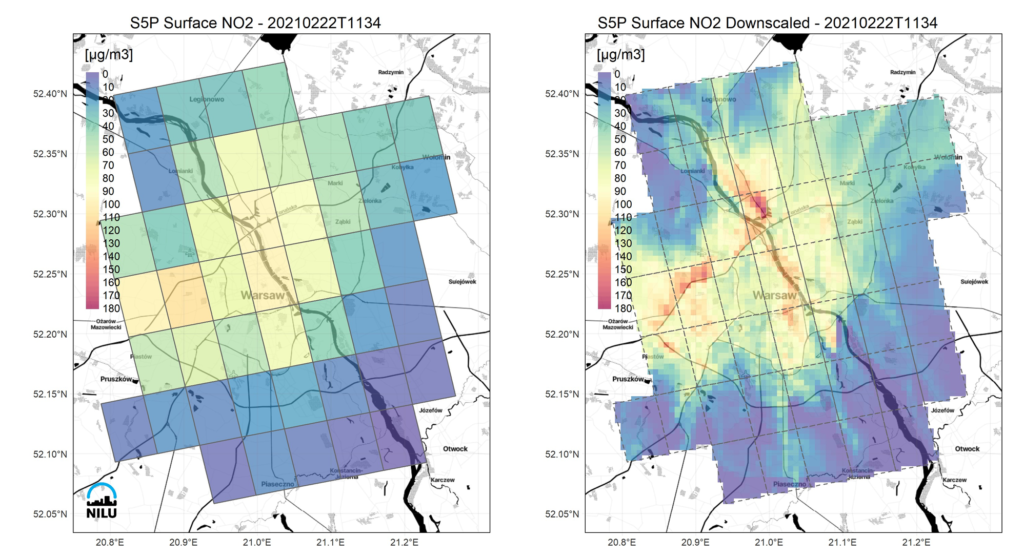